Video
Section CTA
Unlocking revenue diversification: The rising role of MVNOs in Brazil and beyond
Anshul Verma, SVP and Head of Strategic Initiatives at HCLTech, reveals how Mobile Virtual Network Operators will reshape telecom markets worldwide, with Brazil as a key player
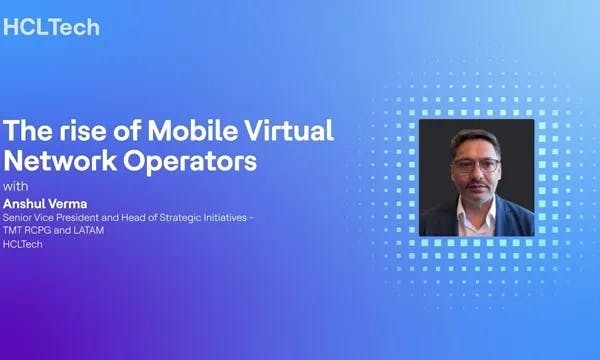
Anshul Verma, SVP and Head of Strategic Initiatives at HCLTech, reveals how Mobile Virtual Network Operators will reshape telecom markets worldwide, with Brazil as a key player
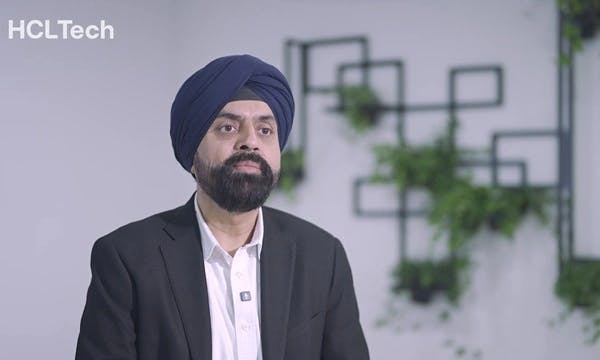
Playing now
Strengthening the digital spine: How telcos can thrive through network transformation
Gurpreet Singh Kohli, SVP, Telco & Enterprise Networks at HCLTech, discusses how telcos can drive growth by modernizing their digital spine with agile tech, innovation and strategic partnerships
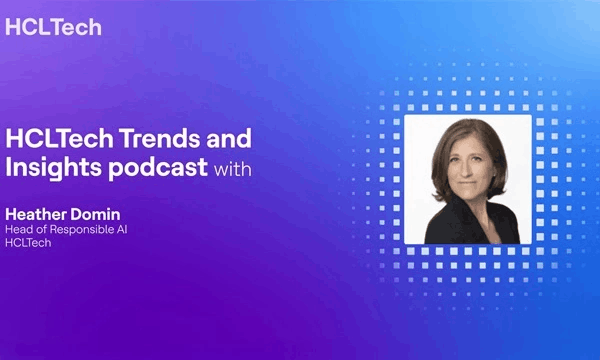
Heather Domin, Head of Responsible AI at HCLTech, joins Nick Ismail on the HCLTech Trends and Insights podcast to discuss why Responsible AI is now viewed as a competitive advantage and how organizations can best implement more ethical standards
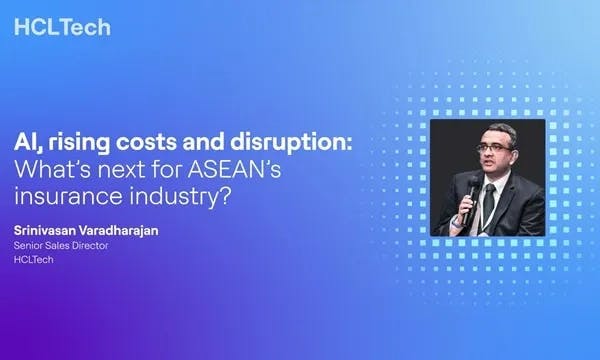
Srinivasan Varadharajan, Senior Sales Director at HCLTech, discusses how AI, rising medical costs and regulatory changes are driving strategic transformation in ASEAN’s insurance industry
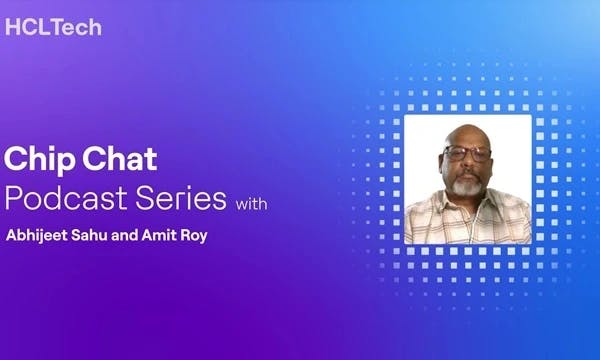
In this Chip Chat podcast episode, Amit Roy highlights how GenAI is revolutionizing various stages of chip design, from initial concept and design to testing and production
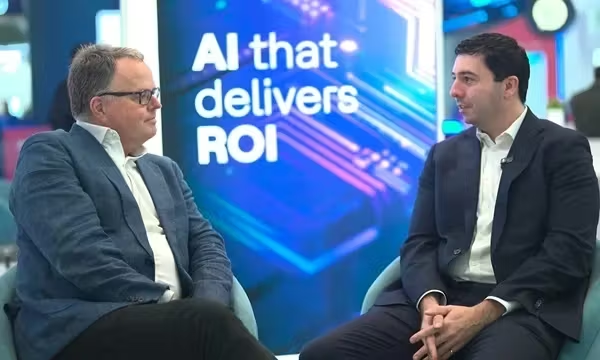
Alan Flower, EVP and Head of AI & Cloud Native Labs at HCLTech, discusses the transformative impact of Agentic AI on customer experience, network management and operations at MWC
Podcast
Section CTA

0:00
progress
00:00
Subscribe to the HCLTech Newsletter
for our latest news and insights