Improving predictive accuracy for a Fortune 50 healthcare conglomerate
Our client, a Fortune 50 healthcare conglomerate, was looking to improve its application to make informed decisions about potential exposure to outdoor allergens. Such applications deliver value to the customer by aiding their assessment of exposure risk, thus reducing their overall symptom burden.
The Challenge
Inadequate metrics
The application relied on limited data and an unmaintained model based on a simple regression algorithm to make predictions. As a result, there was considerable room for improvement in its predictive accuracy. Three key challenges were involved in enabling the application to deliver more value to users:
- The metric for measuring predictive accuracy was inadequate, as it failed to reflect relative class imbalances and the ordering of symptom severity
- Improving predictive accuracy would require adding and preparing new input variables
- Lack of documentation and unavailability of inhouse expertise for the client’s legacy model
The Objective
A significantly improved predictive model
We sought to increase the application’s value to customers and the client alike by improving its predictive accuracy and data utilization potential.

The Solution
Introduced new metrics into the existing model for improved performance
- Improved the quality of feedback that customers received from the application by improving the utilization of existing data sources (pollen count and key symptoms) and expanded the range of sources to weather and patient treatment information
- Reduced the amount of customer input necessary by identifying typical co-occurring symptoms, which made it possible to identify whether the symptoms that the user is experiencing are typically allergic, atypical or mixed regime
- Streamlined future optimization by introducing an optimal metric for measuring the performance of the predictive model used in the application
- The HCLTech team then rebuilt the underlying machine learning model based on a Gradient Boosting Regressor algorithm and changed the programming language from Java to Python
The Impact
Improved product value and user experience
HCLTech delivered the solution in two months. The new data sources and ML model resulted in a consistent 20%-25% uplift in the application’s predictive accuracy. The application made significantly more accurate predictions about likely upcoming symptom severity based on pollen and weather data, as well as symptom and treatment data from the user. The project also paved the way for future developments that will increase the application’s value. Users would benefit from further improvement in predictive accuracy thanks to the addition of air quality data, while the introduction of sales data will enable the indexing of the start of allergy season to help the client find out how it affects the sales of allergy symptom relief products.
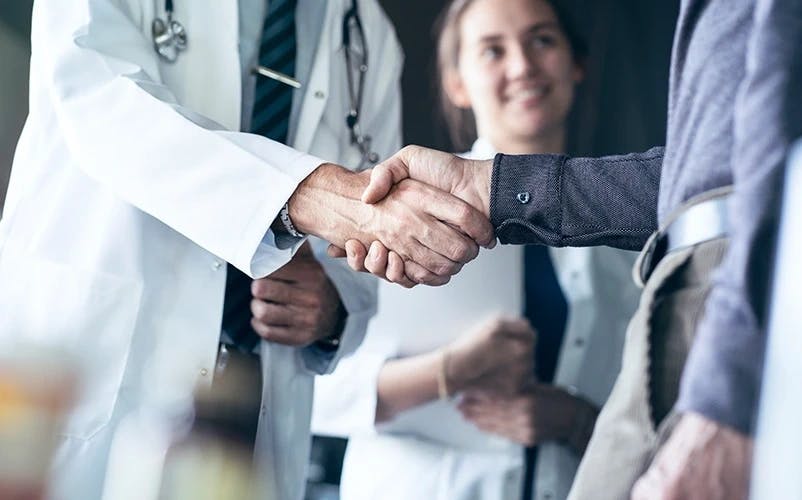