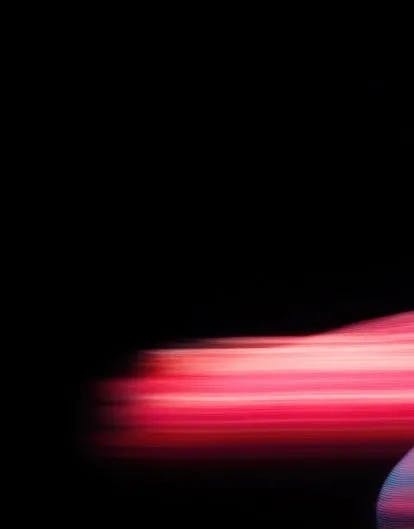
The Blueprint to AI-led Operating Model
Firms are achieving 2x greater customer-centricity through product-aligned operations
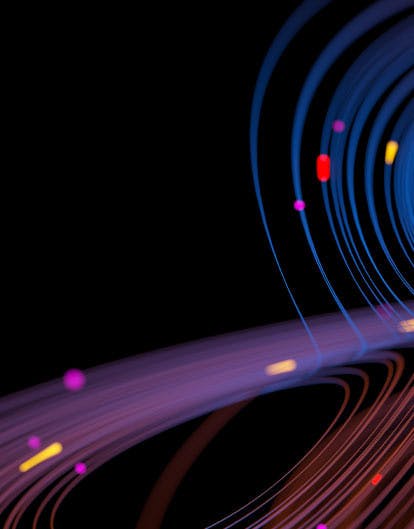
Generative AI’s Impact on Life Sciences and Healthcare
Tech Drives Patient Care Forward
Personalized healthcare. New care delivery options. Ongoing cost pressures against the ever-changing regulatory framework.
Technology is transforming healthcare like never before.
Technological advancements such as telemedicine and AI-driven diagnostics are transforming patient care and disease detection. Genomic research holds promise for personalized medicine while biotechnology can lead to novel therapies.
However, the challenges of affordability, access and data security persist.
Talk to us about modernizing your digital environments and optimizing disparate systems, processes and people.
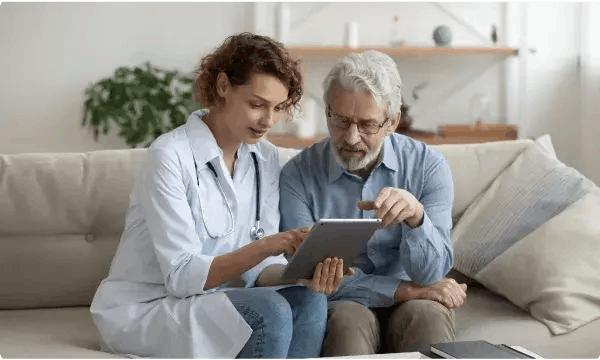
Discover Enterprise Solutions for Life Sciences and Healthcare
Improve your patient care experience, optimize health outcomes and reduce healthcare costs.
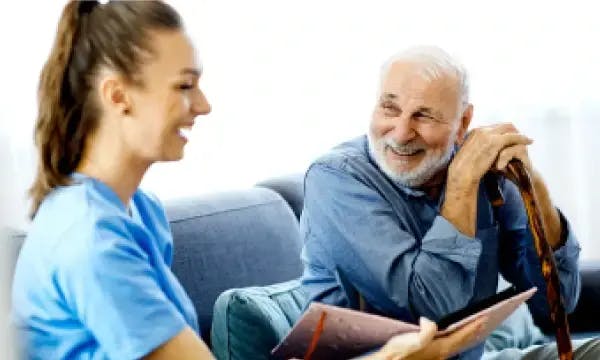
Improve patient health outcomes
Effective care coordination is critical for ensuring quantifiable improvement in patient health outcomes.
HCLTech can help you improve patient intelligence by breaking down siloes of information and providing integrated data and systems.
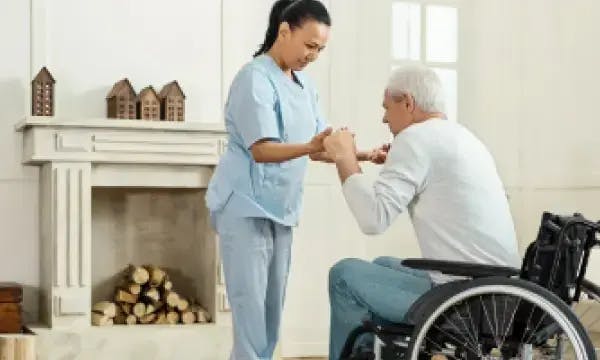
Enhance patient experiences
Enhance your patients' experiences and engagement with digital channels designed to support virtual care and remote healthcare models.
We can help you create secure, simple yet powerful patient portals and telemedicine platforms that will foster greater patient engagement and reduce barriers to care.
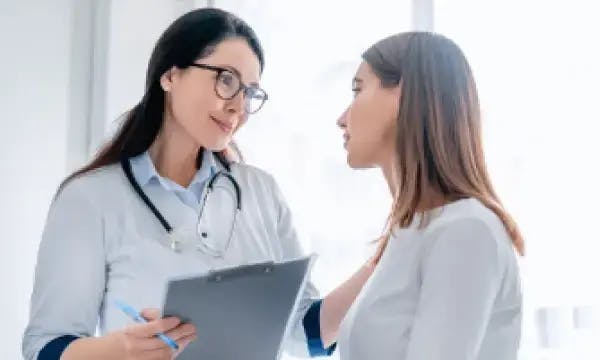
Improve well-being of your staff and care team
Eliminate your staff having to battle with your processes and systems so they can focus their time and energy on doing what they love — providing the best care for their patients.
HCLTech can help you improve staff well-being by modernizing the systems that are necessary for them to do their job without taking time away from patient care.
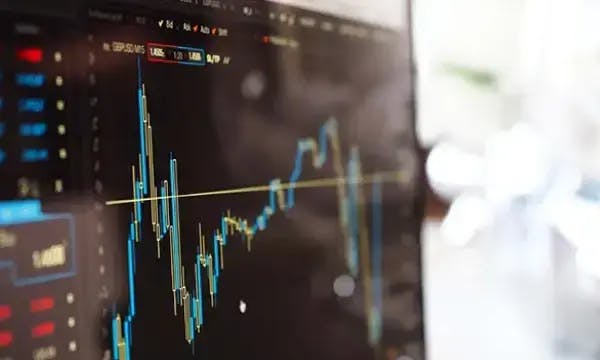
Reduce costs
Understand how to creatively deploy next-gen opportunities for growth by optimizing automation, application modernization and infrastructure improvements to reduce costs and free up resources that can be applied to innovation.
Tap into our decades of life sciences and healthcare experience in helping clients reduce costs with an eye on the ever-changing world of regulations and compliance.
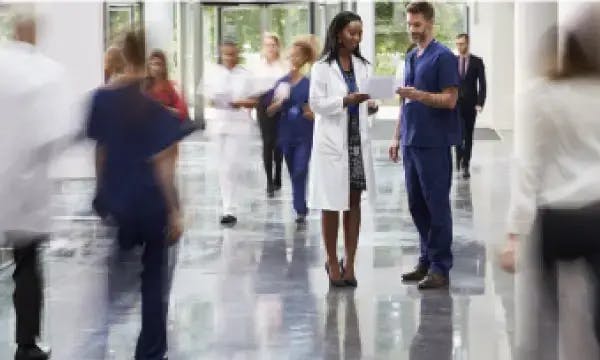
Improve technology investment ROI
HCLTech provides next-gen solutions to modernize your organization’s foundational infrastructure while ensuring compliance and delivering enhanced patient and caregiver experience.
Our experience across a variety of companies within the life science and healthcare space has allowed us to quickly implement, adopt and interconnect new technologies in a way that quickly benefits your organization.
We think these topics might interest you
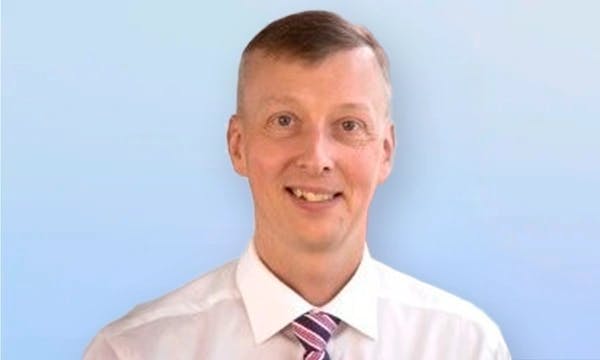
HCLTech has been a dedicated partner to LEO Pharma for over a decade, delivering exceptional global IT support. As LEO Pharma continues its financial turnaround, HCLTech remains a loyal partner, fostering efficiency and innovation.
Our long-standing partnership is deeply-rooted in our trust, collaboration, reliability and a shared commitment to success. We are excited to continue our journey together, unlocking future opportunities for growth and success.
Thomas Lindsted
CIO, LEO Pharma
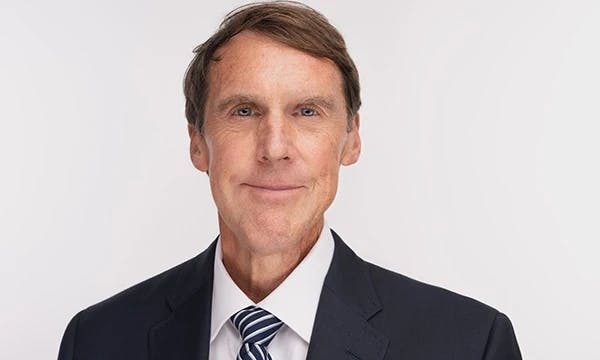
For over the last decade, HCLTech has supported technology services for Syneos Health. Our collaboration, marked by a commitment to quality, reliability and excellence, is now expanding into application services. We’re excited for this new opportunity to work together and look forward to achieving greater innovation, benefits and efficiencies.
Larry Pickett
CIO, Syneos Health
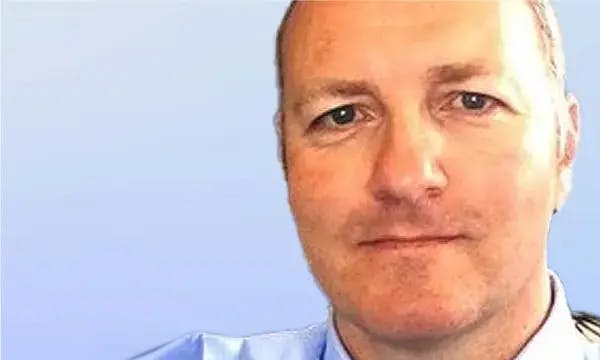
HCLTech has helped us in driving our digital transformation journey. With extensive collaboration and strong and lean governance, we could navigate through periods of change with zero downtime. As one team, we continue to drive digital modernization, which is part of our ongoing strategy to offer digitally enabled services.
Chris Cook
VP, IT Corporate Functions, Smith & Nephew
Driving Value in Life Sciences and Healthcare
Our team has deep expertise in multiple verticals within life sciences and healthcare.
Our mission is to enable a seamless digital transition for your organization while keeping patient outcomes front and center.
Biopharma
Utilize data-driven approaches to enhance clinical trials and leverage technology to accelerate drug discovery and development.
MedTech
Harness technology to innovate medical devices, incorporating sensors and smart connectivity to enable remote patient monitoring and personalization. Employ VR/AR for training and enhanced surgical precision.
Healthcare providers
Streamline administrative tasks and enhance patient care with electronic health records (EHR) systems for efficient data management. Expand patient access to medical services with telemedicine and leverage AI-powered diagnostic tools.
Healthcare payers
Optimize claims processing and fraud detection, employing machine learning algorithms to identify irregularities and ensure accurate reimbursement.
Your Transformation Partner to Help You Reimagine Healthcare
For over 20 years, HCLTech has been a transformation partner for leading and emerging life sciences and healthcare enterprises — always committed to driving unparalleled healthcare experiences across the value chain through:
- Creating unique patient experiences by leveraging domain-specific experience
- Deploying next-gen technology and digital expertise to address the specific needs of life sciences and healthcare
- Meeting the unique regulatory and compliance guidelines for the industry
Empower your life sciences and healthcare enterprise with innovative and industry-leading solutions to grow and transform at scale.
Life sciences companies are our clients
Leading healthcare companies are our clients
Life sciences and healthcare clients around the world
People dedicated to life sciences and healthcare solutions
Success Stories in Life Sciences and Healthcare
Discover how HCLTech helps companies like yours become ready for anything.
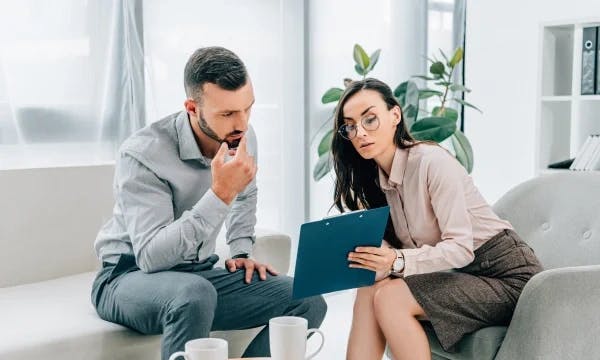
Revolutionizing health plans’ clinical review process with GenAI
HCLTech's GenAI-powered platform transformed a regional Blue Plan's clinical review process, achieving 85% faster reviews and boosting satisfaction for members and providers.
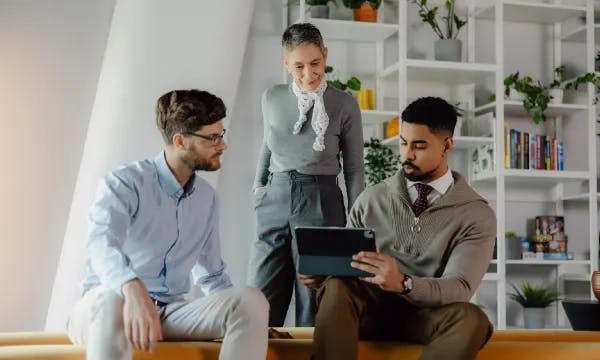
Scaling data with Azure, Databricks, Snowflake and AI for a leading health plan
Discover how HCLTech helped a major US health plan migrate data to the cloud using GenAI, automation, and advanced architecture, boosting performance, user adoption and decision-making.
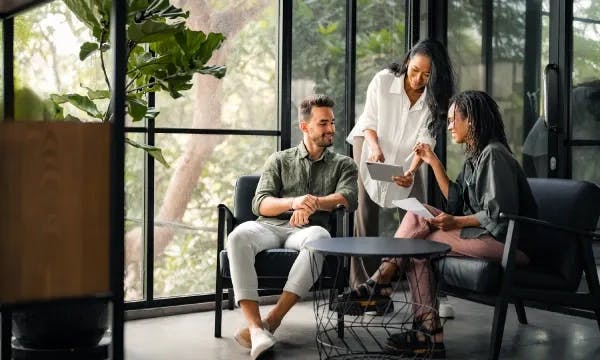
Integrated delivery model helps major health plan thrive in new market dynamics
HCLTech's integrated delivery model revitalized a regional health plan, driving synergy, transformation and efficiency gains while reducing costs and enhancing service delivery.
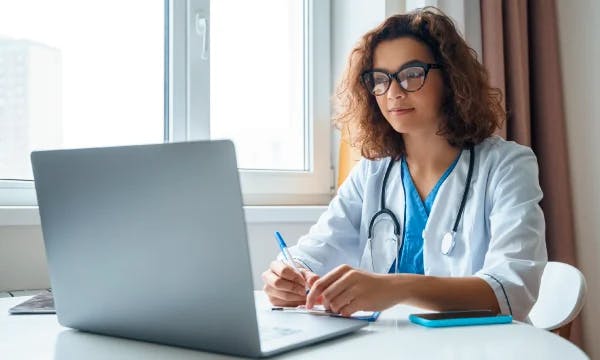
A digital health platform for US veterans
Accelerating digital and cloud-native platforms to address health equity challenge
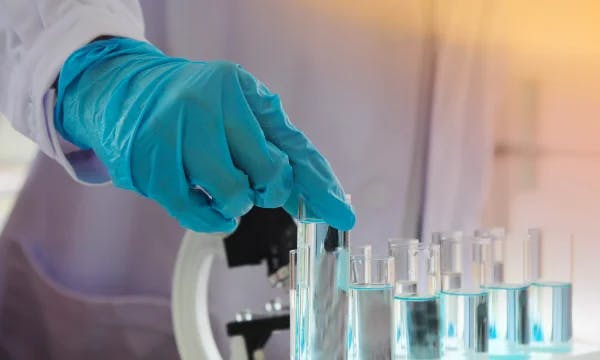
Achieve speed to market with DevOps transformation
Reduced TCO by 35% for a global pharma
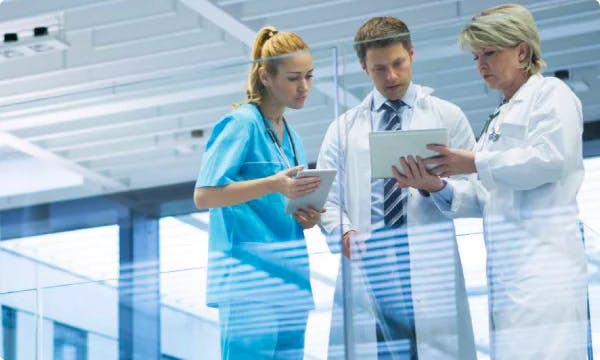
Cloud migration for a leading pharma IT firm
Supporting end users in the cloud and maintaining compliance guidelines
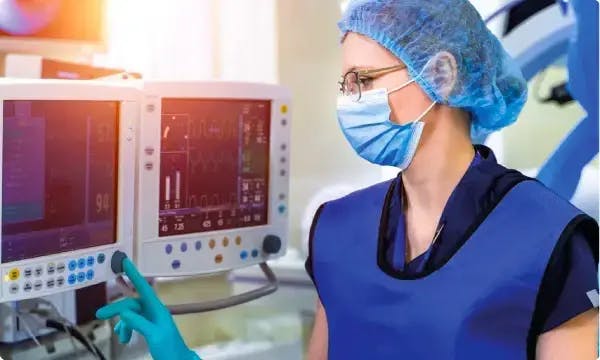
A large healthcare organization adopts One EMR strategy
HCLTech delivered a technology infrastructure to enable a ‘One EMR’ strategy that delivers significant cost savings and improved patient satisfaction.
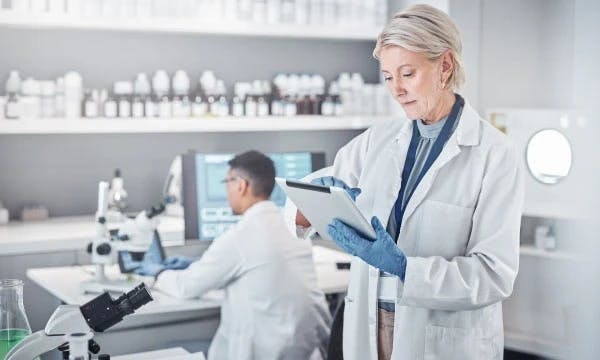
PubMed article summarization tool at a North American Pharmaceutical Company
Revolutionizing efficiency and unlocking 95%-time savings with Generative AI
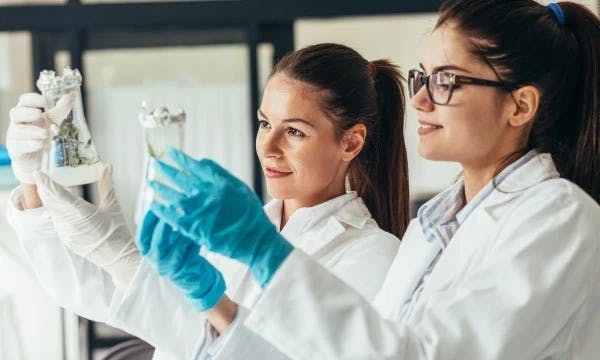
Building rapid innovation GenAl Lab for a Global Pharma Firm
Exploring new horizons of efficiency and reliability with responsible Al
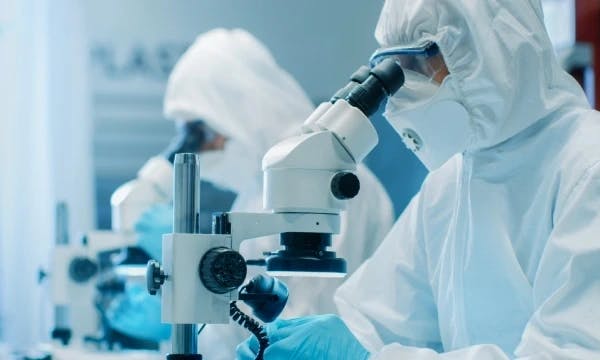
Providing intelligent security to a medical OEM
The client is a US-based company specializing in medical equipment manufacturing and sales of medical devices, instrument systems and reagents.
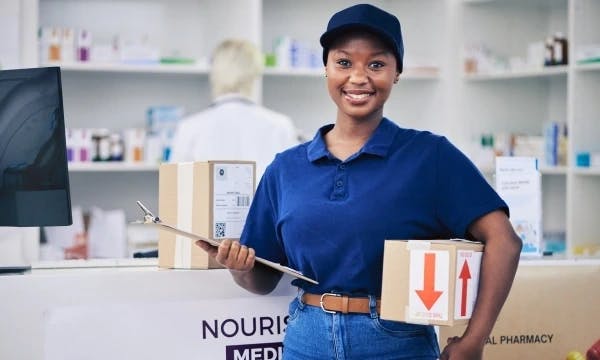
Reducing biopharma supply chain delays to maximize customer satisfaction
We helped a global biopharma leader overcome supply chain disruptions, enhance compliance and cut lead times for enhanced customer satisfaction.
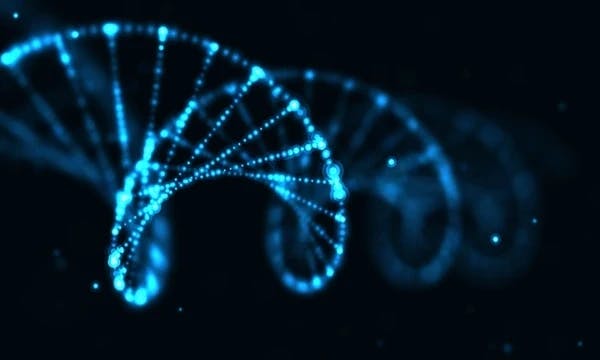
Empowering a major player in life sciences with data modernization on AWS
We delivered cost savings, performance improvement and a future-proof infrastructure for a leading American biopharmaceutical company specializing in drug discovery, clinical trials, etc.
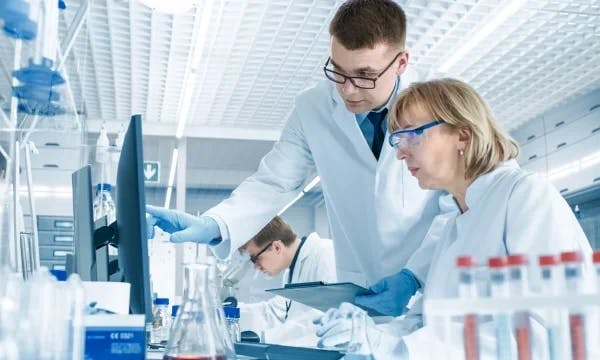
Scaling device distribution for Medidata from 1.5K to 10K+ per month
HCLTech provided a robust delivery model for the client
Making a Name Among Analysts
2023 ISG Provider Lens™ Healthcare Digital Services
HCLTech positioned as a Leader by ISG
2023 Everest Group’s Healthcare Provider Digital Services PEAK Matrix Assessment
HCLTech positioned as a Leader by Everest
2023 Everest Group’s Engineering Services Provider PEAK Matrix Assessment
HCLTech positioned as a Leader by Everest
2022 Everest Group’s Healthcare Payer Digital Services PEAK Matrix Assessment
HCLTech positioned as a Leader by Everest
2022 Everest Group’s Life Sciences Digital Services PEAK Matrix Assessment
HCLTech positioned as a Leader by Everest
HCLTech’s Digital Core
As we have since our beginnings in the 1970s as a plucky tech start-up, we understand that great outcomes begin with a strong foundation of curiosity, knowledge and ingenuity.
Our digital core is built upon the fundamentals of a strong consultative approach, an eye on business outcomes and a drive to supercharge progress for our clients in everything we do.
Powering the future of intelligent insights and data-driven decision-making, our digital and analytics team will help you unlock the full potential of your data, propelling your business forward to a successful future.
Connecting people, processes, things and data, our ‘Define, Build and Run’ offerings and IoT solutions cater to the unique needs of your organization, no matter where you are on your IoT adoption journey.
Elevate the user experience you're delivering, boost uptime and eliminate tech debt with HCLTech’s application services. See around the corner to achieve enterprise-level operational resilience.
DRYiCE™ focuses on leveraging AI and cloud to build products for transforming and simplifying your IT and business operations.
Our infrastructure management services carry some of the most impressive credentials in the IT vendor landscape. We have not only executed complex global IT transformation exercises but also run efficient IT services for over 300 of the world’s leading companies.
HCLTech’s engineering and R&D services combine the technological depth and solution-driven approach required to consistently deliver the results you need in today's competitive landscape. From product blueprinting to support, and from software/hardware to mechanical, we work with over half of the top 100 R&D engineering companies around the world.
HCLTech Digital Process Operations (DPO) digital services and solutions is reimagining the role of technology, from being a driver of marginal efficiency to enabling digital innovation and fueling business performance.
Explore Our Latest Insights into Life Sciences and Healthcare
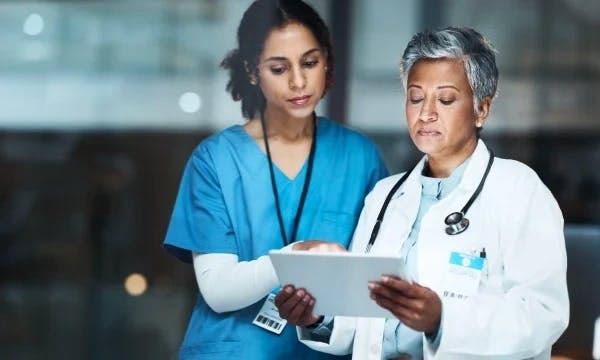
Smart labelling
Discover how our smart labelling solution leverages AI and machine learning to streamline the creation of patient information leaflets, improving efficiency and reducing the processing time.
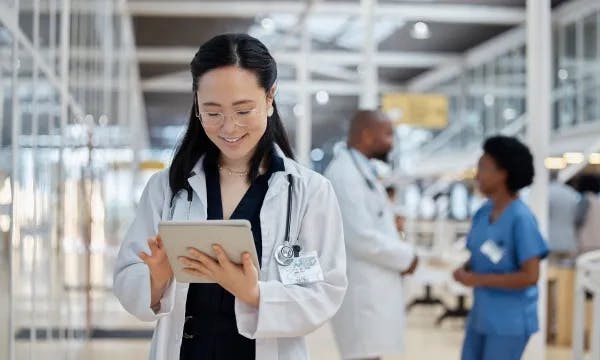
HCLTech Regulatory Intelligence
Unlock the future of compliance with GenAI-powered Regulatory Intelligence, which automates data collection, summarization and reporting to stay ahead of the dynamic regulatory environment.
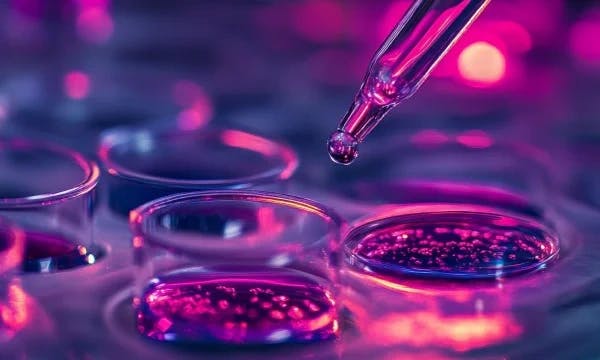
The critical role of REMS measures in ensuring patient safety
Managing the safety profile of pharmaceuticals and medical devices is crucial; comprehensive REMS measures ensure regulatory compliance, mitigate risks and transform patient safety outcomes.
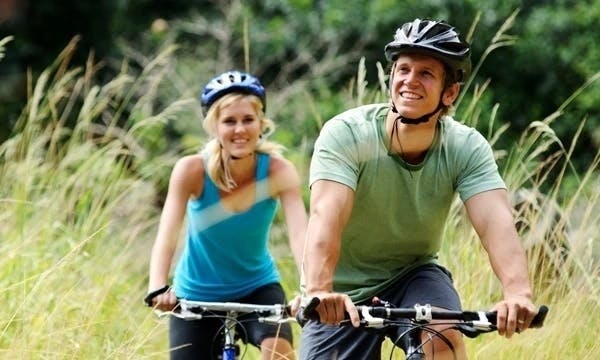
Oriola and HCLTech join hands to enhance customer experience through digital transformation
Oriola Corporation, a leading Nordic health and well-being company, partners with HCLTech to implement SAP S/4HANA utilizing RISE with SAP.
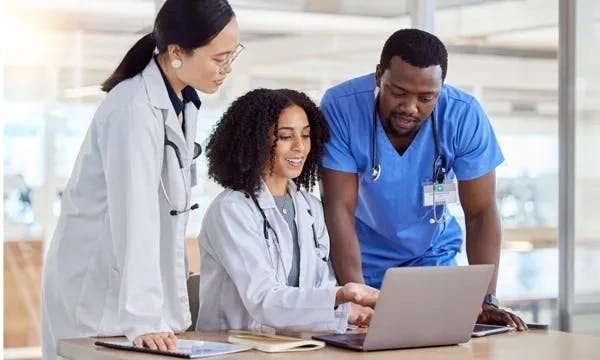
Powering healthcare with 5G
Examining the importance and benefits of 5G in healthcare
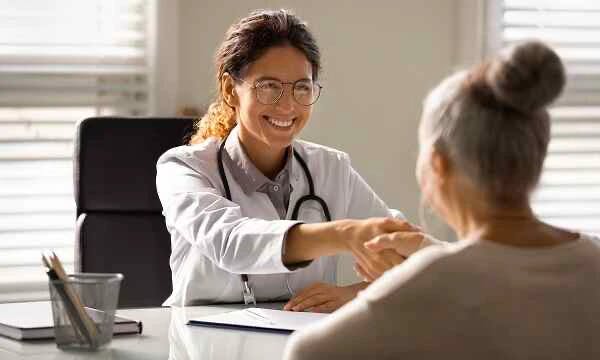
Patient-centric approaches to health data improve data governance
Taking a patient-centric approach to health data governance can help reduce costs, while also improving patient care and gaining new customers
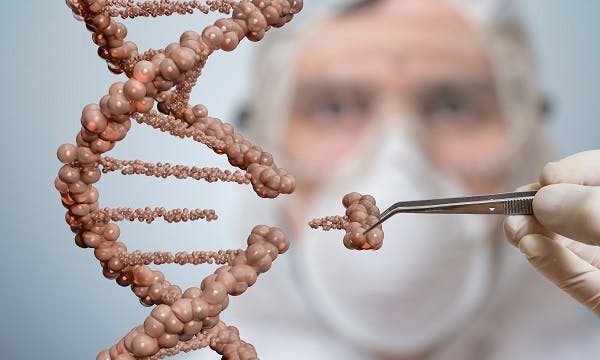
Bioengineering breakthroughs: The rise of organ-on-chip and genome editing
Bioengineering emerges as a dynamic force, weaving precision and personalization into the fabric of a healthier future
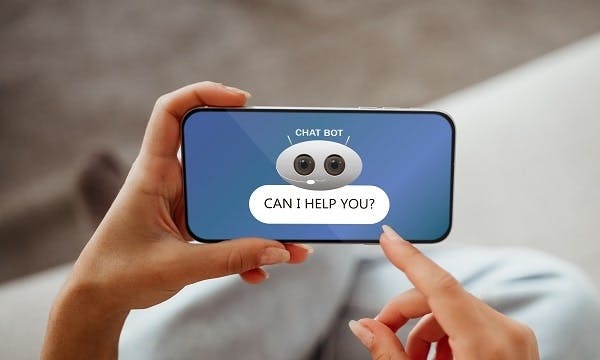
The rise of AI-enabled chatbots in healthcare
Healthcare organizations are turning to AI-enabled chatbots and other emerging AI-enabled technologies to improve patient and customer experience
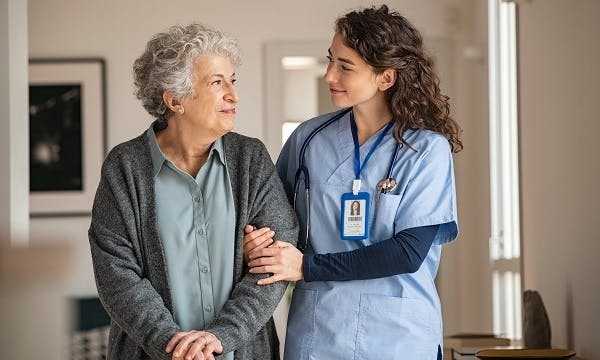
How Total Experience can transform healthcare businesses
The cure for the healthcare distrust epidemic is collaboration. Total Experience connects stakeholders, improves care and rebuilds trust
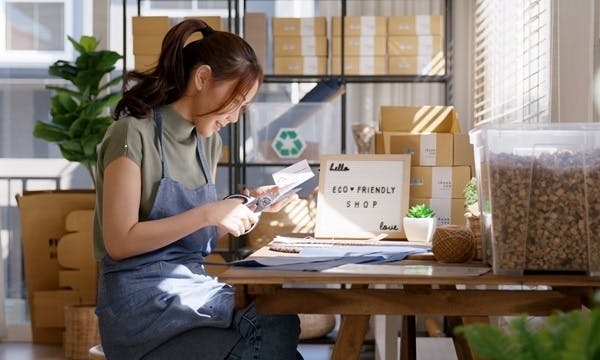
Unlocking sustainability in supply chains: The critical role of data
How to streamline processes and leverage data-driven insights to enhance sustainability within supply chains
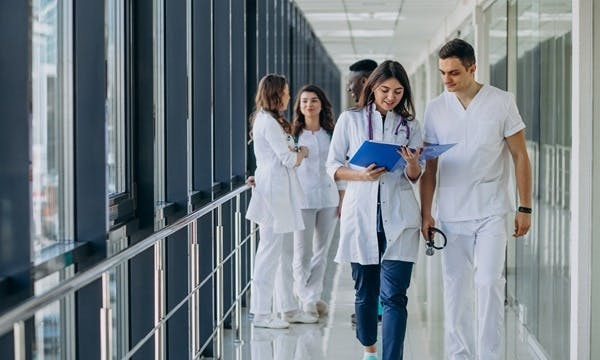
Unveiling the power of real-world data and evidence in healthcare
Discover how real-world data (RWD) and real-world evidence (RWE) are revolutionizing the pharmaceutical industry, reshaping healthcare decision-making and enhancing patient outcomes
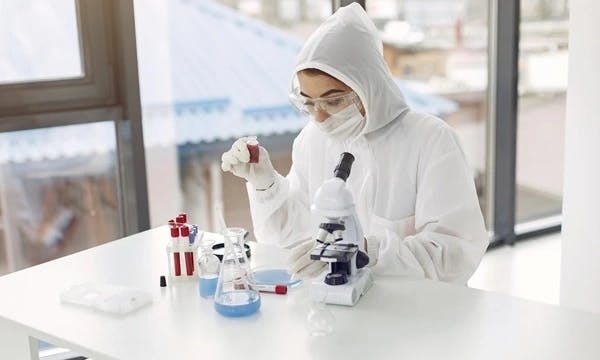
Organ-on-chip technology: The next wave in the healthcare market
What impact will organ-on-chip technology play in the dynamics of the healthcare industry?
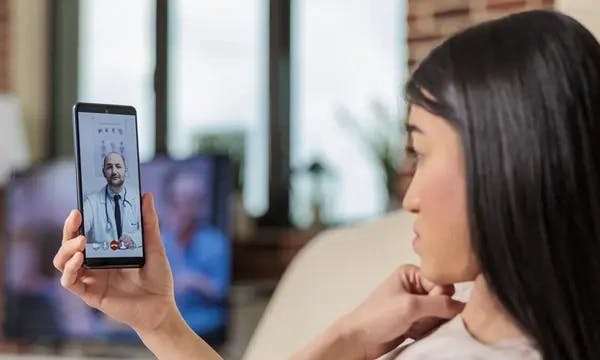
Empowering next-gen healthcare: Integrating digital solutions for patient care
The future of healthcare relies on digital solutions and emerging technologies to deliver exceptional and innovative patient experiences
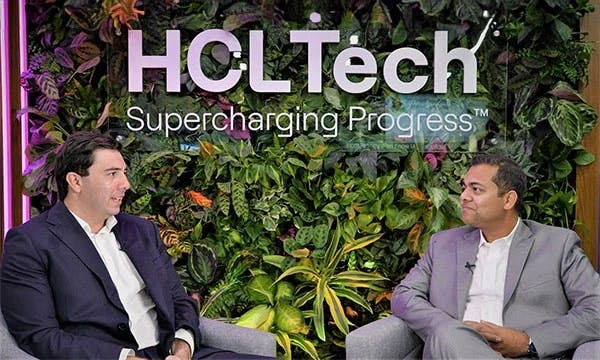
Improving patient experience for a leading biotechnology company
Genmab is working with HCLTech and Salesforce to accelerate digital transformation and improve patient experiences
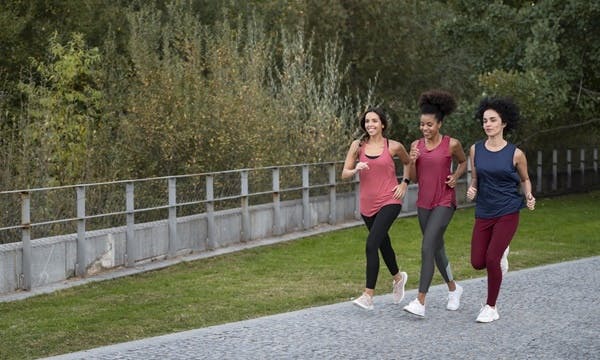
Innovating health, nutrition and beauty: The digital shift at dsm-firmenich
Ipek Ozsuer outlines dsm-firmenich’s commitment to digital transformation, highlighting innovative approaches that prioritize sustainability and ethical practices in a competitive marketplace
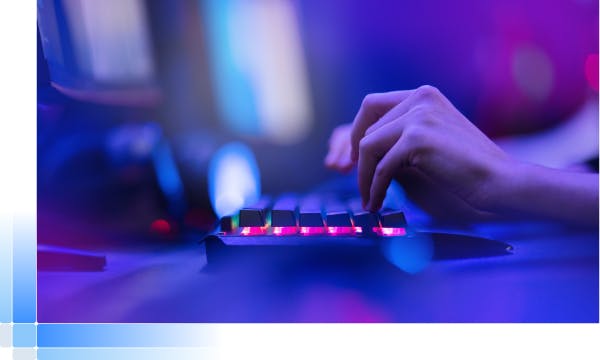
Computer Software Assurance Implementation – A Whitepaper
Download the whitepaper to understand how CSA can enable organizations to achieve higher levels of patient safety, product quality and data integrity.
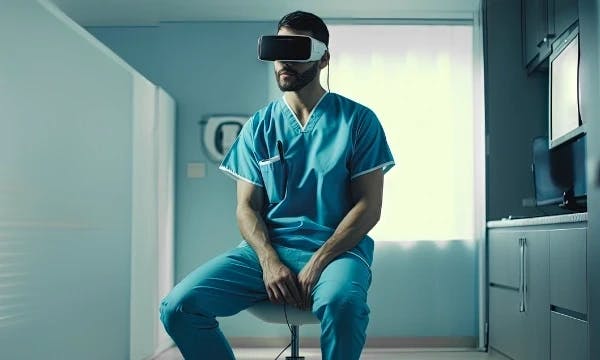
Ready for AI in Healthcare: Evolving beyond hype to enterprise Scale AI
The healthcare industry is constantly evolving, influenced by transformative technologies like GenAI, promising more efficient and effective solutions to healthcare problems.
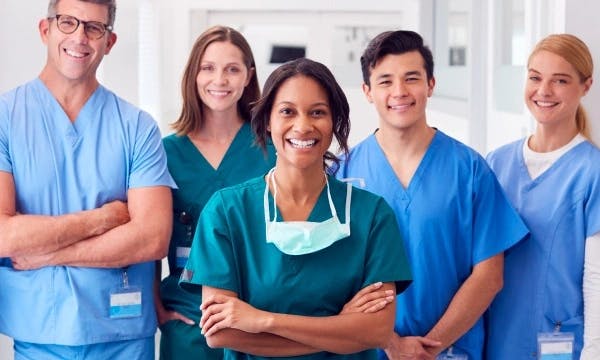
Changing face of prior authorizations
The Prior Authorizations (PA) process has been transformed by integrating AI, ML and GenAI, reducing administrative burdens, enhancing collaboration and achieving cost savings.
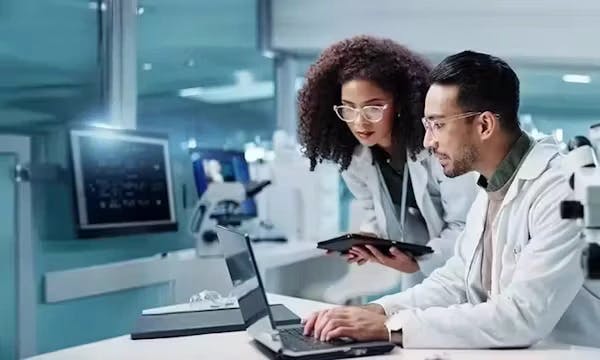
Provider data strategy for sustainable data quality and accuracy
Accurate provider data ensures effective care and reduces costs. HCLTech's data strategy improved accuracy, cut expenses and enhanced engagement for a healthcare organization.
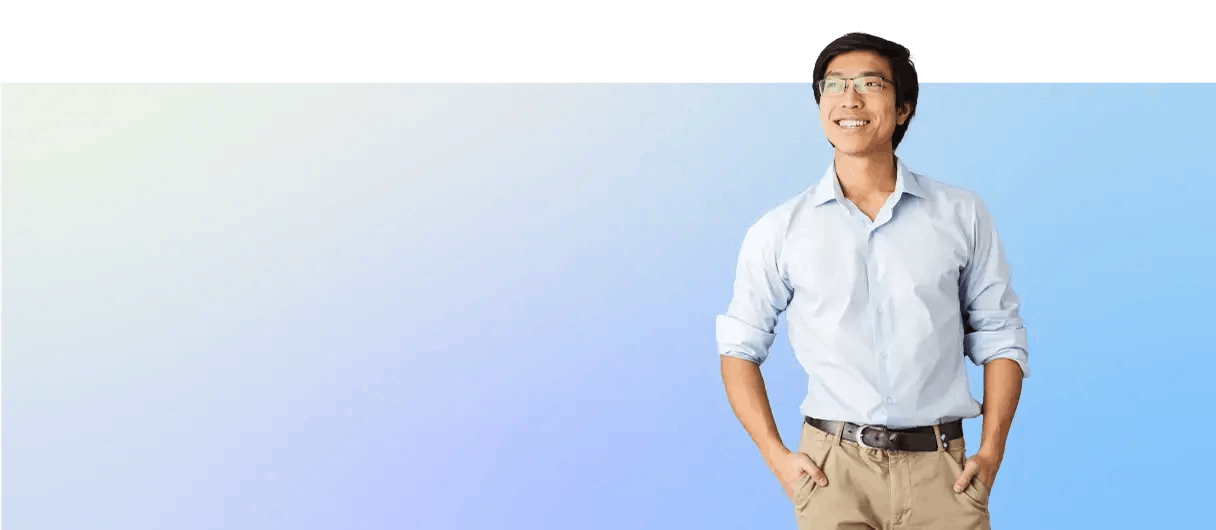
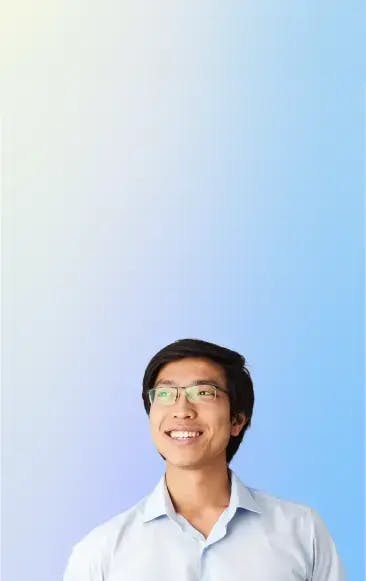
Move Fast. Move Better. Partner with HCLTech.
Take your enterprise to the next level with our life sciences and healthcare solutions.
Subscribe to the HCLTech Newsletter
for our latest news and insights